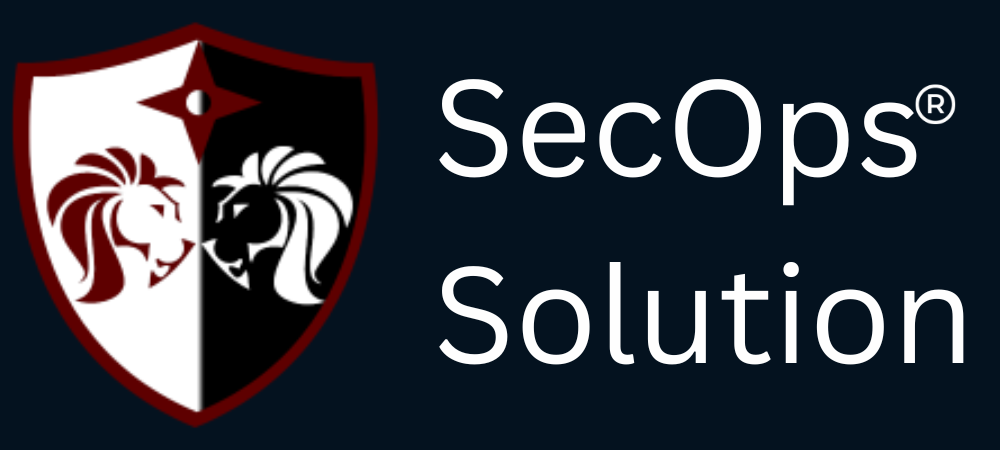
Agentless security for your infrastructure and applications - to build faster, more securely and in a fraction of the operational cost of other solutions

hello@secopsolution.com

+569-231-213
Artificial Intelligence (AI) is revolutionizing various industries, from healthcare and finance to retail and transportation. However, this rapid advancement brings significant concerns regarding data privacy. As AI systems increasingly rely on vast amounts of data to function effectively, understanding the impact of AI on data privacy is crucial. This blog delves into the ways AI influences data privacy, the challenges it presents, and the measures needed to mitigate potential risks.
AI systems, particularly those powered by machine learning (ML) and deep learning algorithms, require substantial amounts of data to train, validate, and optimize their performance. This data often includes personal and sensitive information, such as medical records, financial transactions, and social media activities. The sheer volume and variety of data used in AI applications raise several privacy concerns:
AI algorithms, especially deep learning models, often function as "black boxes," making it challenging to understand how they process and interpret data. This lack of transparency can result in privacy violations without clear accountability.
While data anonymization techniques aim to protect individuals' privacy, they are not foolproof. Sophisticated AI algorithms can sometimes re-identify anonymized data by cross-referencing it with other datasets, compromising privacy.
Obtaining informed consent from individuals whose data is used in AI systems is challenging. Users may not fully understand how their data will be used, leading to potential misuse and privacy violations.
AI systems can inadvertently perpetuate biases present in the training data, leading to discriminatory outcomes. This not only affects the fairness of AI decisions but also raises ethical and privacy concerns for affected individuals.
The regulatory landscape for data privacy is continuously evolving. Ensuring AI systems comply with data protection laws, such as the General Data Protection Regulation (GDPR) and the California Consumer Privacy Act (CCPA), is a complex and ongoing challenge.
Incorporating privacy principles into the design and development of AI systems is essential. This approach, known as Privacy by Design, ensures that privacy considerations are integrated from the outset, rather than being an afterthought.
Developing explainable AI models can enhance transparency and accountability. By providing insights into how AI algorithms make decisions, organizations can build trust and address privacy concerns more effectively.
Implementing advanced data anonymization techniques can help protect individuals' privacy. Combining anonymization with techniques such as differential privacy can further enhance data protection.
Enhancing informed consent mechanisms ensures that individuals understand how their data will be used. Clear and concise privacy notices, along with user-friendly consent options, can help achieve this goal.
Regularly monitoring and auditing AI systems for privacy compliance is crucial. This includes assessing data handling practices, evaluating algorithmic fairness, and ensuring adherence to regulatory requirements.
Collaboration among stakeholders, including policymakers, researchers, and industry leaders, is vital for addressing AI-related privacy challenges. Joint efforts can lead to the development of standardized guidelines and best practices for AI and data privacy.
The impact of AI on data privacy is profound and multifaceted. While AI has the potential to drive innovation and improve various aspects of life, it also poses significant privacy risks. By understanding these challenges and implementing robust privacy measures, organizations can harness the power of AI while safeguarding individuals' privacy rights. As AI continues to evolve, a proactive and collaborative approach to data privacy will be essential to building trust and ensuring ethical AI practices.
SecOps Solution is a Full-stack Patch and Vulnerability Management Platform that helps organizations identify, prioritize, and remediate security vulnerabilities and misconfigurations in seconds.
To learn more, get in touch.